Our leading-edge software is designed to do marketing analytics better, quicker and cheaper. Our packages include econometrics, machine learning, marketing spend optimisation, market structure analysis and price & promotional analysis.
Econometrics

Econometrics is one of the key analytical techniques used in Marketing Mix Modelling (MMM). However, traditional approaches typically involve considerable effort in model development and report preparation, the net effect being to push up the cost per model produced.
For this reason, we have developed a software package which is designed specifically to make the use of econometrics in marketing as streamlined and efficient as possible. Our StatsPro software contains a range of standard regression models including Ordinary Least Squares, Logit & Probit, and Bayesian Inference. What makes it different is the range of special productivity features that enable it to deliver well-formed MMM models quickly and cheaply, as follows:
- A quick and easy method of including, omitting or fixing variables.
- The user can experiment with lagged variables, differences, logs, dummies, trends etc. without re-defining the model.
- Built-in functionality to enable diminishing returns curves and stocked variables to be included in linear regression models.
- The ability to generate new variables “on-the-fly” without having to calculate separate data series.
- Automated production of reports and charts on predicted versus actual values, residuals, decomposition and waterfalls for all model types, including log and difference models.
- A highly intuitive and visual approach to system models
- Optional automated inclusion or exclusion of variables based on the coefficient, p-value or t-stat.
- The option to switch seamlessly to Bayesian inference (either totally or partially), if required.
Machine Learning
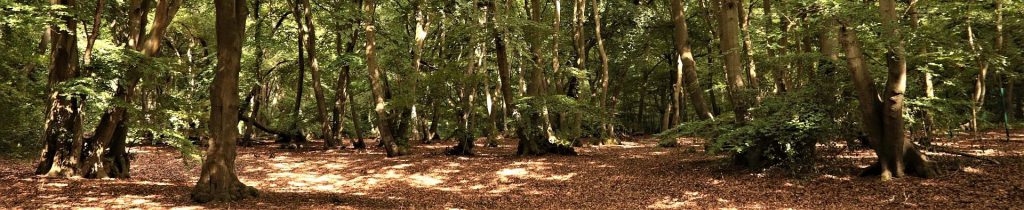
The latest addition to our software portfolio is the ForestPro Machine Learning (ML) package. This provides an easy-to-use implementation of the main Random Forest algorithms.
However, what is different about ForestPro is that it is designed to be user-friendly for MMM. It provides all of the same functionality as conventional econometric packages such as StatsPro and, in particular, it provides:
- Fully automated sales decompositions, which can be used to estimate the Returns on Investment (RoIs) for media spend.
- Saturation curves for media variables – including the coefficients required as inputs for media spend optimisation.
- An easy way of handling system models and also problems in which the dependent variable is binary.
- And – it is available (in addition to other implementations) as an Add-In to Excel, and can be used with no knowledge of programming languages such as Python.
So, what do the results look like? And how do they differ from those that you would get from a conventional MMM approach using OLS? Well, superficially, the results look quite similar. However, comparing MMM examples across a range of sectors leads to the following general conclusions about the Machine Learning approach:
- It generally produces a model which provides a better description of the data, and often without the need for dummy variables.
- Machine Learning can often provide separate estimates for the media variables in situations where OLS can only estimate aggregated variables.
- And it identifies non-linear and synergistic effects and automatically includes these in the structure of the model. For example – if sometimes (but not always) media and promotions were run contemporaneously, this will be taken into account. Similar considerations apply to competitor activity.
Overall, and most importantly, ForestPro provides a quicker and easier way of producing good MMM models than the conventional OLS approach – and does not require specialised knowledge of or skills in econometrics.
Market Structure

Econometric analysis has deservedly acquired a reputation of being one of the cornerstone analytical tools in the field of marketing. However, when adequate data are not available for econometric analysis, the question arises – what alternative approaches are available? Market Structure Analysis attempts to fill this gap by making estimates of consumer responses from limited data about the brands or products competing in the market.
An early type of this analysis was pioneered by the Hendry Corporation, but is subject to some limitations. For this reason, Quantalise developed its own innovative approach to Market Structure Analysis. Each brand is regarded as a member of a set of brands with which it competes, to a greater or lesser extent. By default, the system will estimate the degree of competition within this “partially-competing set” automatically from price positions and distribution data. But, optionally, if direct information is available on the competitive structure, then this can be substituted.
Typically, the data required to carry out Market Structure Analysis comprise representative annual data for all of the major competing brands for sales volume, sales value, and media spend. Optionally, if available, data on margins or distribution can be used to improve the estimates.
From these data, the software constructs a map of the competitive interactions and uses this to estimate for each brand the response curve of incremental sales to media spends, and also the optimum media spend for each brand.
Optimisation

The OptPro marketing spend software tool helps businesses manage their marketing spends quickly, easily and effectively. It optimises the allocation of spend across products, brands, regions, media channels, and over time – and, if required, all of the above in one optimisation model!
So – the questions that OptPro helps to answer include the following:
- How much should I be spending on which brands and in which regions?
- What will be the impact on sales and profit contribution of optimising marketing spends?
- What is the cost to the business of placing constraints on the levels of marketing spend?
- How do I choose the media mix that maximises the overall reach at a specified frequency?
- What is the optimum laydown for my media activity over time?
The main inputs required are the media response curves that describe how sales respond to the various types of media activity. These data can be obtained either from econometric analysis (Ordinary Linear Regression, Bayesian Inference or Machine Learning) or from Market Structure Analysis.
OptPro contains a highly efficient proprietary calculation algorithm based on an advanced mathematical approach. As a result, it provides virtually instantaneous answers in almost all situations. The software is quick and easy to use and is highly scalable to handle large and complex problems for a wide variety of scenarios and portfolios.
Pricing

Our PriceMax software is designed to inform pricing strategy by enabling the impact of “what-if” pricing scenarios on sales volumes, sales revenues and market shares to be evaluated rigorously and quickly. These scenarios can include sets of price changes for both our brands (or SKUs) and competitor brands (or SKUs).
The software does this by estimating the full set of cross price elasticities across all brands (or SKUs) in the category, using a proprietary algorithm based on switching theory which:
- Ensures that the changes in the average category sales volume and average category price are consistent with the specified market price elasticity for any given price change or set of price changes.
- Estimates the degree of competition between the brands (or SKUs) in the category.
The inputs required are the sales values, sales volumes and own price elasticities for the competing brands (or SKUs), together with the market price elasticity. The estimates of the price elasticities can be obtained either from econometric analysis or market structure analysis (see above).
Promotions
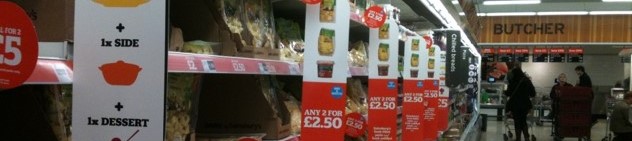
The PromoMax software is an interactive business planning tool that is designed to help companies decide when they are doing the right thing in terms of category, brand, product, channel, customer, feature, price and promotional strategy. For example, it helps answer questions such as:
- Price
- Which SKUs are under-priced, and which are over-priced?
- How does our price-pack architecture fit in with the market and compare with that of competitors?
- Promotions
- Which promotional mechanics or in-store executions work for which products and channels?
- Are promotions profitable for both manufacturers and retailers?
- How does our promotional strategy compare with that of our competitors?
The input data required are:
- Sales data: Retailer sales data on value and volume, by SKU, retailer and week.
- SKU data: For example, SKU size, brand and manufacturer. Also, for your “own” SKUs, the cost of goods and trade price.
- Promo data: (optional) event data by SKU, start date and end date, and trade rebates.
In summary, PromoMax is a fact-based, decision-making system designed to inform strategy on price and promotions. The system automates the processing of data from various sources and then makes the results available in the Microsoft Power BI data visualisation tool and, if required, Excel spread sheets.